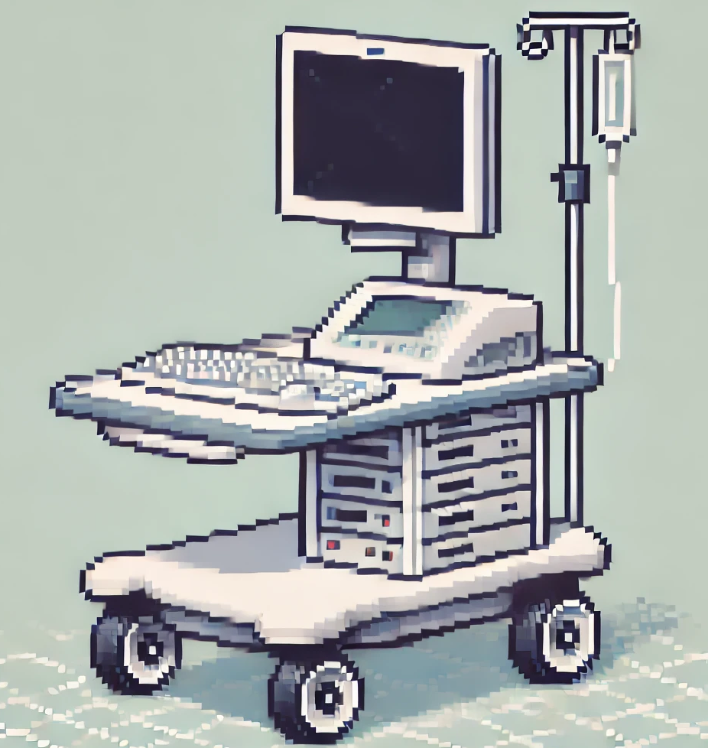
How Racial Bias in Lab Testing Impacts AI in Healthcare
Artificial intelligence (AI) is transforming healthcare, but what happens when the data feeding these powerful tools carries the weight of systemic inequities? A recent study highlights how racial disparities in emergency department (ED) laboratory testing could reinforce biases in AI models, potentially widening the health equity gap. If you’re working to make healthcare more just, this is a critical issue you can’t ignore.
This research found significant differences in how often Black and White patients received common lab tests during ED visits. These discrepancies—rooted in systemic inequities—aren’t just numbers on a chart. They reveal patterns of undertesting that could undermine the fairness of AI-based tools, which increasingly guide clinical decisions. Let’s unpack the study’s findings, their broader implications, and the urgent call to action they inspire.
Racial Disparities in Lab Testing: A Wake-Up Call
The study, conducted at two U.S. teaching hospitals, compared lab test rates for Black and White patients in the ED. Researchers used a matched cohort design to control for factors like age, sex, chief complaints, and ED triage scores. Despite these adjustments, stark disparities persisted:
- Complete Blood Count (CBC): White patients were 1.7–2.0% more likely to receive this test, a cornerstone of diagnostic decision-making.
- Metabolic Panel: White patients were tested 1.5–1.9% more often.
- Blood Cultures: Again, White patients had higher testing rates (0.7–0.9%).
Interestingly, Black patients were slightly more likely to receive tests like troponin, used to diagnose heart issues. But even this difference doesn’t negate the broader pattern of inequities in care delivery.
These differences aren’t just academic. They highlight how Black patients may receive less diagnostic scrutiny, which could result in missed diagnoses and inadequate care. For AI models trained on this skewed data, the implications are chilling. If these tools “learn” from biased patterns, they risk perpetuating the very inequities they’re meant to solve.
Why It Matters for Public Health and AI
AI holds incredible promise in healthcare. From predicting sepsis to optimizing resource allocation, these tools have the potential to save lives. But their power lies in their data. When biases like those revealed in this study are baked into the training datasets, the resulting AI systems can amplify harm rather than reduce it.
Here’s how it happens:
- Biased Training Data: Many AI models assume that untested patients have normal results. When testing rates differ by race, this assumption can skew risk predictions. For example, Black patients may appear less likely to have certain conditions simply because they weren’t tested as often.
- Spurious Correlations: AI might “learn” that being Black correlates with lower risk for conditions diagnosed via lab tests, even when this isn’t true.
- Unequal Outcomes: In practice, this could mean fewer resources or less aggressive treatment plans for Black patients, reinforcing existing disparities.
This isn’t just a technical issue—it’s a moral one. Public health practitioners and researchers must act to ensure that AI doesn’t deepen the very inequities it aims to address.
The findings align closely with the Robert Wood Johnson Foundation’s JUSTICE SQUARED initiative, particularly Shift 2: Removing racism from clinical, operational, and administrative processes. JUSTICE SQUARED challenges healthcare organizations to:
- Measure and account for racism—not just race—in diagnostics and algorithms.
- Eliminate practices that embed racial bias into healthcare processes.
This study serves as an example of the need for action. By uncovering how racial testing differences could bias AI, it reinforces the need for systemic change in how data is collected, analyzed, and applied.
What Can Be Done?
Transforming these insights into action requires a multifaceted approach:
- Audit and Adjust Algorithms: Healthcare organizations must evaluate whether their AI systems disproportionately disadvantage racial minorities. Models should be re-trained or recalibrated to mitigate bias.
- Reimagine Testing Protocols: Clinicians need to examine how implicit biases influence decisions about which patients receive tests. Standardized protocols can help ensure equitable care.
- Collaborate with Communities: True equity starts with listening. Healthcare systems should partner with affected communities to co-design solutions that address systemic barriers.
- Build Equitable Governance: Establish committees with diverse representation to oversee the implementation of AI and ensure transparency in decision-making.
- Invest in Structural Change: Programs like JUSTICE SQUARED provide a blueprint for organizations to root out systemic racism in healthcare processes, including diagnostics and resource allocation.
Join the Conversation
What do you think about these findings? Have you seen similar patterns of inequity in your work or community? Share your thoughts in the comments or join us on social media to discuss how we can advance racially just healthcare together.
Be Part of the Change – Get Weekly Updates!
Stay informed and connected. Subscribe for free and share this blog to make a difference in public health with others. If you liked this blog, please share it! Your referrals help This Week in Public Health reach new readers.