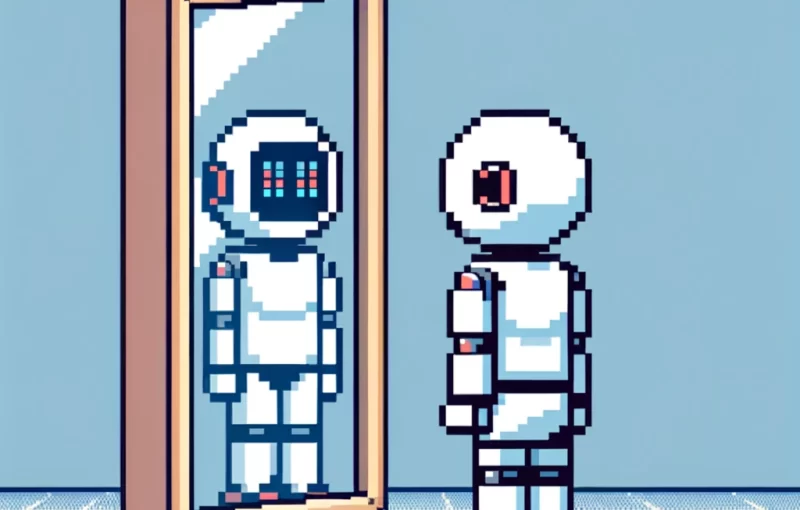
Unraveling the Enigma of AI Bias in Healthcare: A Call to Action for Public Health Practitioners
by Jon Scaccia April 1, 2024The emergence of artificial intelligence (AI) in healthcare promises a revolution in patient care and treatment outcomes. However, the insightful article Bias in artificial intelligence algorithms and recommendations for mitigation, highlights a critical issue: the inherent bias in AI algorithms. This piece aims to dissect the complex layers of this bias and its implications for public health practitioners.
Understanding the Bias in AI
Artificial Intelligence (AI) in healthcare represents a cutting-edge frontier where technology meets medicine. It’s designed to enhance diagnostic accuracy, inform treatment planning, and predict patient outcomes with precision. However, beneath this technological marvel lies a critical issue: the prevalence of bias. These biases aren’t always overt; they subtly infiltrate various stages of AI algorithm development, leading to discrepancies in healthcare delivery. It’s essential to peel back these layers to understand how and where these biases originate.
The Genesis of Bias: From Development to Deployment
The journey of an AI algorithm in healthcare begins with its development. Here, the initial framing of the problem sets the stage. Often, the issues chosen for AI intervention are those recognized and understood by the developers, who may not represent the full spectrum of the patient population. This lack of diverse perspectives at the inception can skew the algorithm’s focus, inadvertently sidelining less recognized but equally critical health issues prevalent in marginalized communities.
As we progress to data collection, the biases become more pronounced. AI algorithms learn from data, but what if the data itself is biased? Healthcare datasets often reflect historical inequalities: underrepresentation of certain demographic groups, biased treatment practices, and uneven documentation of health conditions. When algorithms are trained on such data, they inevitably inherit these biases. For example, an algorithm trained predominantly on data from one ethnic group may be less accurate in diagnosing conditions in people from a different ethnic background. This misalignment between training data and real-world applications can lead to misdiagnoses or inappropriate treatment recommendations, disproportionately affecting marginalized groups.
The Peril of Processing and Interpretation
The next critical phase is data processing and interpretation, a stage ripe for bias to further entrench itself. Algorithms are coded to interpret and process data in specific ways. This process, though technical, is not immune to human prejudices. Decisions made in data preprocessing, such as which variables to include and how to handle missing data, can introduce subtle biases that alter the algorithm’s outcomes. For instance, if certain symptoms are more prevalent in a specific gender or race and are not adequately represented in the dataset, the algorithm may overlook these symptoms, leading to a gender or race-based diagnostic bias.
The final stage, model implementation, is where these biases manifest in clinical settings. Even a well-designed AI tool, when deployed in a diverse healthcare environment, can exhibit unforeseen biases due to differences in patient populations, healthcare practices, or data environments. For public health practitioners, this is the critical point where the theoretical limitations of AI meet the practical realities of patient care. It’s where the bias embedded in algorithms can translate into real-world disparities in healthcare delivery.
The bias in AI in healthcare is a multifaceted issue that permeates every stage of algorithm development and deployment. From the initial problem framing to the final implementation, each step carries the potential to introduce or perpetuate biases. These biases ultimately impact the effectiveness and equity of healthcare delivery, making it crucial for developers, healthcare providers, and policy makers to collaboratively address these challenges at every stage.
The Impact on Public Health Practice
For public health professionals, understanding and addressing AI bias is crucial. It affects health equity, access to care, and the quality of medical services offered to diverse populations. The article emphasizes several potential steps to mitigate AI bias.
- Inclusive Problem Framing: The journey to bias mitigation in AI starts with how problems are defined. A diverse team must ensure the inclusion of varied perspectives right from the outset.
- Diverse Data Collection: AI algorithms must be trained on data sets representing different demographics. This broadens the scope and applicability of AI in healthcare.
- Ethical Data Preprocessing: Transparent preprocessing strategies that recognize and adjust for biases in data are vital. This includes handling missing data and outliers sensibly.
- Conscientious Model Development and Validation: Models should be developed and validated with the awareness of potential biases. They must be tested in diverse settings to ensure their reliability across different patient populations.
- Responsible Model Implementation: Post-implementation monitoring is essential to catch and correct biases that may become evident only in real-world settings.
Implications for Public Health Practitioners
Public health practitioners play a pivotal role in mitigating AI bias. They must:
- Advocate for inclusive and ethical AI development practices.
- Promote education and awareness about AI biases within healthcare settings.
- Collaborate with AI developers to ensure the representation of diverse populations in AI models.
- Ensure continuous monitoring and assessment of AI tools in practice to identify and rectify biases.
Conclusion: A Call for Collaborative Action
Addressing AI bias in healthcare is not just a technical challenge but a societal imperative. Public health practitioners, alongside AI researchers, policymakers, and healthcare providers, must work collaboratively. The goal? To harness AI’s power to enhance, not compromise, health equity.
Empower Your Public Health Journey – Subscribe and Transform!
Unlock the transformative power of knowledge with ‘This Week in Public Health.’ Each issue is a treasure trove of insights into crucial research, community health achievements, and advocacy strategies. Don’t just read about change – be the agent of it. Subscribe for free and start making an impact with each edition!
Leave a Reply