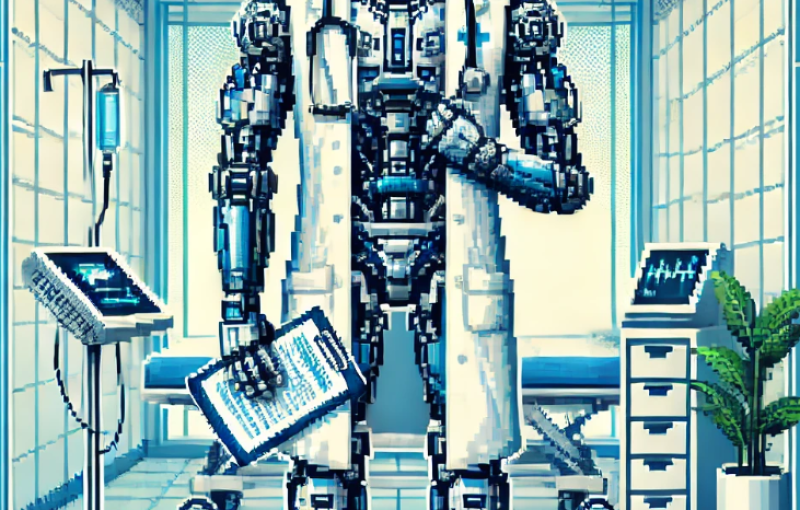
The Economic Ripple Effect of Rare Diseases: How Artificial Intelligence Can Change the Narrative
by Jon Scaccia January 16, 2025Imagine the frustration of living with a mystery illness for years. Your symptoms worsen, your work performance declines, and your income takes a nosedive. All the while, you shuttle between doctors, waiting for a definitive diagnosis that feels perpetually out of reach. For millions of people worldwide with rare diseases, this isn’t a hypothetical scenario—it’s their daily reality.
Rare diseases, defined as affecting fewer than 1 in 2,000 people, represent a daunting challenge for public health systems. With over 300 million people affected globally, these conditions collectively present significant social and economic burdens. However, a recent study suggests that advances in artificial intelligence (AI), particularly diagnostic decision support systems (DDSS), could offer a lifeline to patients and alleviate some of these burdens. Here’s how.
A Long Road to Answers
Rare diseases are notoriously difficult to diagnose. Patients wait an average of 4 to 5 years for a correct diagnosis. Misdiagnoses and unnecessary treatments are common, adding to the emotional, physical, and financial toll. Beyond the personal impact, these delays create ripple effects that affect workplaces and economies.
The study conducted at Hannover Medical School analyzed the economic impact of delayed diagnosis in 71 patients with rare inflammatory systemic diseases. Using the WHO’s Health and Work Performance Questionnaire (HPQ), researchers tracked work performance and income at four key points: the onset of symptoms, the hypothetical time when a DDSS could have suggested the correct diagnosis, the actual diagnosis, and the time under proper therapy.
The Economic Toll of Rare Diseases
The findings are stark: patients lost an average of 5.32% of their monthly income during their diagnostic odyssey due to work absenteeism and decreased productivity. The losses were even more pronounced for patients with joint, muscle, and connective tissue involvement compared to those with vascular diseases. Early retirement compounded these challenges, with most patients retiring nearly two decades before the statutory retirement age, resulting in a collective net income loss of €13 million.
These numbers are more than just statistics—they represent missed opportunities, stalled careers, and the erosion of financial stability. For many, the journey to a correct diagnosis feels like a race against time, with every delay exacerbating the fallout.
How AI Could Rewrite This Story
Enter diagnostic decision support systems like Ada DX. By analyzing patient symptoms and test results, these AI-driven systems generate probable diagnoses, helping clinicians navigate complex cases. In the study, Ada DX could have identified the correct diagnosis for 42 of the patients earlier than traditional methods. This earlier intervention could have reduced average income losses from 5.32% to just 2.66%.
The implications are profound. By shortening the diagnostic journey, DDSS tools can reduce the economic impact of rare diseases, allowing patients to return to work sooner or avoid unnecessary medical expenses. For employers, this translates into higher productivity and lower absenteeism, creating a win-win scenario.
Beyond the Numbers: The Human Impact
Behind every data point is a story of resilience and struggle. Consider Anna, a fictionalized composite of patients in the study. Anna began experiencing debilitating joint pain in her early 30s. Over the next five years, she visited multiple specialists, undergoing countless tests, all while her ability to work declined. By the time she received a diagnosis of a rare connective tissue disorder, her income had halved, and she was considering early retirement.
Now imagine a scenario where Anna’s doctor used Ada DX. Within months of her first symptoms, the system flagged her condition as a top diagnostic possibility. Early treatment enabled Anna to manage her symptoms effectively, allowing her to continue working and maintain her financial independence.
This isn’t just about preventing economic loss—it’s about preserving dignity and giving people their lives back.
Barriers and Future Directions
While the potential of DDSS tools is promising, there are hurdles to widespread adoption. Integrating these systems into clinical workflows requires investment, training, and trust from healthcare providers. Additionally, diagnostic tools must account for the diversity of rare diseases and patient presentations to be truly effective.
Future research should focus on quantifying the broader societal benefits of these systems, including their impact on healthcare costs, patient quality of life, and caregiver burden. Policymakers and healthcare organizations must also address structural barriers, such as long waiting times for specialist appointments, to maximize the benefits of AI.
Join the Conversation
How can public health systems encourage the adoption of AI tools like DDSS? What policies or initiatives could bridge the gap between technology and patient care? Have you or someone you know faced challenges with a rare disease diagnosis? Share your thoughts and experiences in the comments or on social media using #AIForRareDiseases. Together, we can drive awareness and advocate for solutions that make a difference.
Join the Community – Get Your Weekly Public Health Update!
Be a health leader! Subscribe for free and share this blog to shape the future of public health together. If you liked this blog, please share it! Your referrals help This Week in Public Health reach new readers.
This is a fascinating look at how AI could revolutionize rare disease diagnosis. What do you think are the biggest barriers to getting these tools widely adopted in healthcare, and how can healthcare systems overcome them?