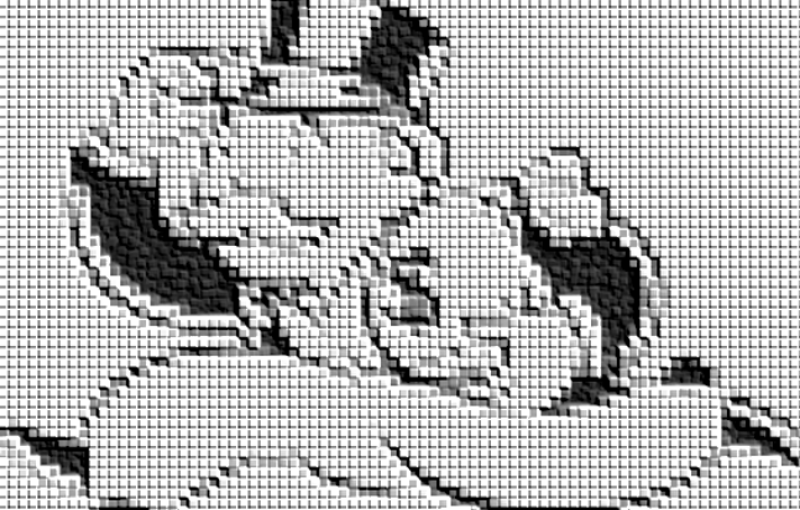
Data Integrity in a Divided World: The Smart Case for Inclusivity
by Mandy Morgan February 14, 2025The current socio-political climate has made it dangerous to engage in equity work.
The federal government’s attacks on diversity, equity, and inclusion (DEI) initiatives have created an environment where organizations must rethink how they communicate about equity without compromising core values. While the language of fairness and justice is under siege, the principles behind equity—ensuring accuracy, efficiency, and effectiveness—remain critical for economic competitiveness and institutional trust.
I recently wrote about some linguistic changes that honor the letter of the executive orders, if not the spirit. I have no idea if these would actually be successful, so let’s change strategies.
One way to bridge the ideological divide is by framing equity not as a political stance but as a matter of data integrity. Incomplete, biased, or misleading data leads to inefficient decision-making, wasted resources, and poor business outcomes. Ensuring that data is comprehensive and representative benefits not only marginalized groups but also businesses, policymakers, and the workforce at large.
Why Accuracy in Data Matters
Avoiding Costly Mistakes
Businesses and policymakers rely on data to make strategic choices, yet when that data is incomplete or skewed, it leads to bad decisions. For example, workforce training programs based on outdated labor statistics have resulted in job seekers being trained for roles that no longer exist, leading to wasted funds and economic stagnation. Similarly, companies that use flawed customer data to inform marketing campaigns often misallocate their budgets and fail to reach key consumer segments. If I’m trying to run a vaping cessation campaign for teens, why would I spend ad dollars on Linkedin? Tiktok or Instagram would be more meaningful.
Enhancing Predictive Power
The ability to forecast trends and anticipate risks is only as strong as the data-feeding predictive models. If decision-makers fail to incorporate diverse data sources, they risk basing their strategies on an incomplete picture of the market. In business, this can result in product failures, misaligned supply chains, and unaddressed consumer needs. In public health, it can lead to ineffective medical interventions that do not account for real-world population diversity.
Building Public Trust in Institutions
Trust in institutions is eroding, and misinformation thrives in environments where data integrity is questioned. Organizations that demonstrate transparency in their data collection and decision-making processes foster credibility among consumers, employees, and the public. When people see that data reflects a full, accurate representation of reality, they are more likely to engage with and support policies and initiatives.
The Costs of Bias in Data
Impact on Business Efficiency
Companies that rely on narrow or biased datasets risk making strategic mistakes that lead to inefficiencies. Bias in data occurs when certain groups or perspectives are underrepresented, overrepresented, or misrepresented, leading to skewed results that distort decision-making and create inefficiencies.
A hiring algorithm that excludes certain demographic groups not only raises ethical concerns but also limits access to top talent, weakening an organization’s competitive edge. Similarly, biased medical data leads to treatments that fail to meet the needs of entire patient populations, reducing the efficacy of healthcare interventions. Also thinking of a poll that relies on land lines.
Economic Consequences of Flawed Data
Data bias doesn’t just impact individuals—it carries measurable economic costs. Poor data quality can lead to:
- Lower revenue due to ineffective marketing strategies.
- Operational inefficiencies due to misallocated resources.
- Missed opportunities in emerging markets due to inadequate insights.
For example, credit scoring models that fail to account for alternative financial histories have historically prevented qualified individuals from accessing loans. When financial institutions corrected for these gaps, they expanded their customer base, increased lending revenue, and strengthened economic participation. If we put our capitalist hat on, this is a good thing!
How Comprehensive Data Improves Decision-Making
Expanding the Data Pool for Better Insights
By incorporating a wider range of data sources, businesses and policymakers can identify hidden trends that would otherwise be overlooked. Consumer spending patterns across diverse demographics provide a more accurate representation of market demand, allowing for more targeted and effective decision-making.
Mitigating Unintentional Blind Spots
Many organizations collect data from a narrow or homogeneous group, leading to skewed results. Broadening data collection efforts improves the validity of decision-making models. For example, clinical trials historically relied on data from a limited patient demographic, resulting in ineffective treatments for broader populations. By expanding research samples, pharmaceutical companies have developed more effective drugs with fewer side effects, improving health outcomes for all. Again, this is good thing!
Improving Workforce and Productivity
Organizations that prioritize inclusive and representative data practices create stronger, more efficient teams. By identifying the best talent and optimizing operational workflows, companies enhance productivity and innovation, leading to long-term success.
Practical Steps for Strengthening Data Integrity
Best Practices in Data Collection and Analysis
- Ensure diverse data sources: Expand datasets to capture a broader range of insights.
- Validate data accuracy: Regularly audit data sources for reliability.
- Reduce algorithmic biases: Implement fairness testing in AI-driven decision models. This has been discussed in many sources. I’m particularly partial to Cathy O’Neil’s Weapons of Math Destruction.
Technological and Policy Solutions
- Adopting AI-driven data validation tools to detect errors in real-time.
- Establishing industry-wide standards for data collection and reporting.
- Encouraging transparent data-sharing practices among businesses and research institutions.
Conclusion: The Path Forward
DEI initiatives are under attack.
Organizations must rethink how they advocate for equity. By framing equity as a data integrity issue rather than a social justice one, we can appeal to a broader audience and emphasize the business and economic benefits of comprehensive, accurate, and representative data.
The future of data-informed decision-making depends on it.
Time is Running Out – Join the Public Health Movement Now!
Get trusted insights that help you drive change in your community. Subscribe to our free newsletter today and stay informed.
🔥 Your action matters—share this blog right now to grow our network of changemakers!
Leave a Reply