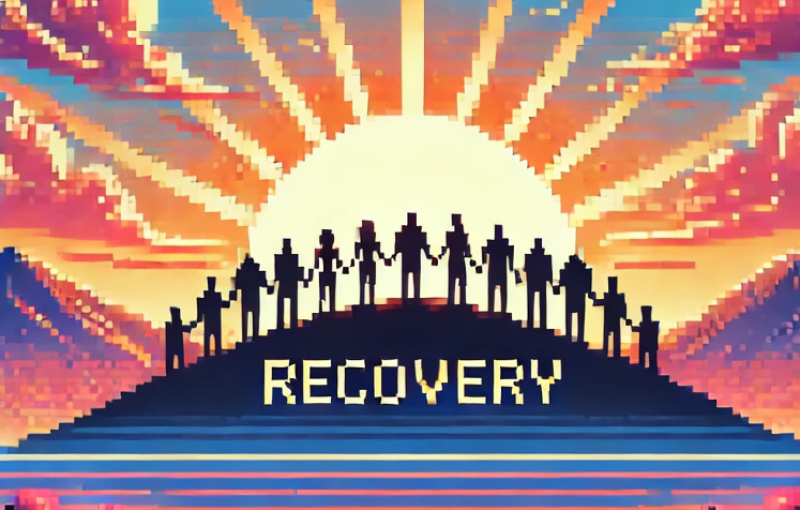
What If We Could Predict the Perfect Treatment for Opioid Use Disorder?
by Jon Scaccia December 24, 2024Imagine a future where every person struggling with opioid use disorder (OUD) could be matched with the treatment most likely to work for them—saving lives, reducing relapses, and offering hope to thousands of families. Achieving this vision has long been a challenge for clinicians, but researchers behind a groundbreaking study at the U, Columbia, and City College of NY have taken a major step forward.
Their mission? To harmonize data from three large-scale clinical trials on medication-assisted treatment (MAT) for OUD. While the science of MAT has proven effective, the ability to tailor treatment to individual needs has been hindered by disorganized, incompatible data. This blog delves into the painstaking process of harmonizing disparate datasets and why it holds such promise for the future of addiction treatment.
Why This Research Matters
The opioid epidemic has wreaked havoc across the U.S., with overdose deaths nearing 100,000 annually. Despite effective treatments like methadone, buprenorphine, and extended-release naltrexone (XR-NTX), matching the right treatment to the right person remains elusive. The problem isn’t a lack of data but a lack of usable data. Clinical trials often operate in silos, using different methods, instruments, and definitions that make their results difficult to compare.
Enter the CTN-0094 project, led by researchers working with the National Drug Abuse Treatment Clinical Trials Network (CTN). By harmonizing data from three major trials, they created a robust resource that allows for more nuanced analyses of patient characteristics, treatment responses, and outcomes.
The Data Challenge: Chaos Before Clarity
Let’s break this down with an analogy. Picture three sprawling libraries, each containing books on the same subject—but with no standardized cataloging system. One uses the Dewey Decimal system, another organizes books by color, and the third by the author’s zodiac sign. Finding patterns across these libraries would be maddening.
The researchers faced a similar scenario. The three trials—CTN-0027, CTN-0030, and CTN-0051—varied in how they defined variables like substance use, withdrawal symptoms, and even basic demographic information. For instance:
- Substance use: One trial lumped all opioids together, while another separated heroin from prescription opioids.
- Demographics: Race and ethnicity categories differed, with one trial offering detailed subcategories while another used broad labels like “Other.”
- Withdrawal scales: Some trials used clinician-reported scores, while others relied on self-assessments, making direct comparisons impossible without harmonization.
Harmonization in Action
To tackle these challenges, the team employed a meticulous, multi-step approach:
- Standardizing Variables: They aligned data from over 1,500 survey items across the three trials, creating consistent definitions for variables like “opioid use days” and “severe withdrawal symptoms.”
- Building a Unified Database: The harmonized data now lives in a tidy, relational database with 23 interconnected tables. This structure eliminates redundancy and simplifies analysis.
- Double-Helix Approach: Two independent teams reviewed and cleaned the data, ensuring accuracy and minimizing biases.
The result? A dataset covering over 3,500 individuals, complete with detailed documentation to guide future research.
Key Findings: Who Seeks Treatment for OUD?
The harmonized data revealed striking insights into the diverse population seeking treatment for OUD:
- Age: Patients ranged widely in age, but CTN-0027 included an older subset, likely reflecting differences in recruitment settings.
- Gender: Women were better represented in CTN-0030, which focused on prescription opioid users—a group with higher female prevalence.
- Substance Use Patterns: CTN-0027 and CTN-0051 predominantly enrolled heroin users, while CTN-0030 focused on those misusing prescription opioids.
These differences underscore the importance of tailoring treatment approaches to the unique needs of subgroups within the broader OUD population.
What’s Next?
The harmonized dataset is a treasure trove for researchers, but the work is far from over. Future efforts could focus on:
- Predictive Models: Using the data to identify which patient characteristics best predict treatment success.
- Expanding Harmonization: Applying these methods to other substance use disorders and datasets, including electronic medical records.
- Standardizing Data Collection: Ensuring future trials adopt consistent, harmonized protocols from the outset.
Lessons for Public Health Practitioners
For those working in public health, this research offers several actionable insights:
- Invest in Data Infrastructure: The harmonization process highlighted how better-designed data systems could save time and resources while improving outcomes.
- Prioritize Diversity: Pragmatic trials like these, which include diverse populations and real-world settings, provide a more accurate picture of treatment effectiveness.
- Collaborate Across Disciplines: The project’s success hinged on expertise from biostatistics, psychiatry, addiction medicine, and data science—a reminder of the power of interdisciplinary collaboration.
Join the Conversation
What excites you most about the potential of harmonized data to improve addiction treatment?
- How could these findings influence your work in public health, clinical care, or policy?
- What challenges do you foresee in scaling up these methods?
Share your thoughts in the comments or join us on social media. Let’s keep the conversation going!
By transforming fragmented data into a cohesive whole, the CTN-0094 project has laid the groundwork for more personalized and effective OUD treatments. This isn’t just about data—it’s about saving lives.
Explore the Data.
Find the github for this research at this link.
Join the Community – Get Your Weekly Public Health Update!
Be a health leader! Subscribe for free and share this blog to shape the future of public health together. If you liked this blog, please share it! Your referrals help This Week in Public Health reach new readers.
Leave a Reply