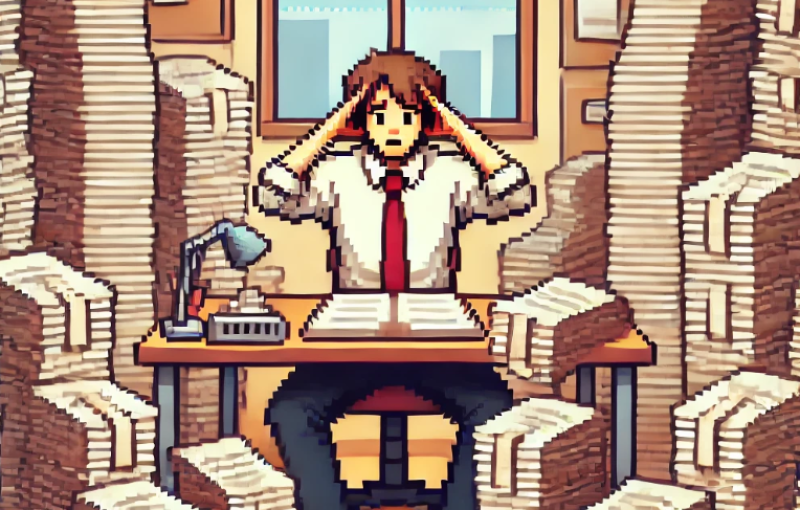
Best Practices for Meta-Analysis: The Key to Stronger, More Reliable Research
by Jon Scaccia October 1, 2024When we think about gathering scientific knowledge, many of us picture researchers conducting experiments, analyzing their results, and drawing conclusions. But what if we want to go beyond individual studies to understand the bigger picture? This is where meta-analysis comes in. A meta-analysis is a method that allows researchers to combine the results of multiple studies, offering a broader view of the evidence on a given topic. It’s a powerful tool for answering important questions in public health, psychology, management, and many other fields.
However, as with any tool, how well it works depends on how you use it. The strength of a meta-analysis hinges on how exhaustive the search for relevant studies is and how accurately those studies are coded and interpreted. Unfortunately, many meta-analyses fall short in these areas, leading to weaker conclusions. This post will explore the key findings of a recent paper on best practices for conducting meta-analyses in organizational psychology and management, but the lessons can apply to any field.
Why Meta-Analyses Matter
In public health and related fields, decision-makers often rely on evidence from meta-analyses to guide policies and programs. A well-conducted meta-analysis can help us understand what works, for whom, and under what conditions. For example, a public health official might use meta-analysis data to decide which interventions are most effective at reducing obesity or improving mental health. But if the studies included in the meta-analysis are poorly selected or coded, the results may be misleading.
The goal of a meta-analysis is to generalize findings across multiple studies, providing a more comprehensive understanding than any single study can offer. However, the strength of a meta-analysis lies in the quantity and quality of the studies it includes. If a meta-analysis overlooks key studies or misinterprets them, the conclusions drawn can be flawed. As the old adage says, “garbage in, garbage out.”
The Importance of Exhaustive Searching
One of the main challenges of conducting a meta-analysis is ensuring that the search for relevant studies is exhaustive. This means casting a wide net to include as many relevant studies as possible, not just those that are easy to find.
Imagine you’re a fisherman trying to catch enough fish to feed your village. If you only fish in one small pond, you might catch a few fish, but you’ll likely miss out on the bigger, tastier fish in the ocean. Similarly, if a meta-analyst only searches a few well-known databases, they may miss important studies published in less prominent journals or in other languages.
Unfortunately, many meta-analyses do not search thoroughly enough. They may only include studies from top-tier journals or studies published in English, leading to biased results. For example, studies from lower-income countries, where public health challenges may differ, are often underrepresented in meta-analyses. This lack of diversity in the data can skew the results and reduce the generalizability of the findings.
To avoid this, the authors recommend using multiple databases, including those that cater to underrepresented populations or fields. They also suggest partnering with a librarian or bibliometrician, who can help identify harder-to-find sources of data.
Accurate Coding: Avoiding Apples and Oranges
Once relevant studies have been identified, they must be coded accurately. Coding involves categorizing the studies based on their variables, outcomes, and other important details. The goal is to ensure that similar studies are compared to each other, rather than lumping together studies that measure different things.
For example, in a meta-analysis of workplace stress, you wouldn’t want to combine studies measuring job satisfaction with those measuring burnout, even though both are related to workplace stress. Mixing “apples and oranges” in this way can lead to misleading conclusions.
The authors highlight the importance of training coders to ensure consistency and accuracy. They also recommend using a tiered coding process, where studies are first screened based on broad criteria (e.g., whether they are empirical) and then more closely examined for specific details (e.g., the variables they measure). This helps reduce the risk of coding errors and ensures that only the most relevant studies are included in the final analysis.
The Dangers of Garbage In, Garbage Out (GIGO)
Even if a search is exhaustive, a meta-analysis can still suffer from poor-quality data if the studies included are not representative or if they are incorrectly coded. This is where the concept of “garbage in, garbage out” comes into play. If the studies fed into the meta-analysis are biased or flawed, the results will be equally unreliable.
For example, if a meta-analysis only includes studies from top-tier journals, it may suffer from publication bias. This occurs when studies with positive results are more likely to be published than those with negative or inconclusive findings. As a result, the meta-analysis may overestimate the effectiveness of an intervention.
Similarly, if a meta-analysis only includes studies conducted in wealthy, Western countries, it may not accurately reflect how an intervention would work in lower-income settings. To avoid this, the authors recommend being mindful of inclusion criteria and ensuring that the studies selected are as representative as possible.
Practical Takeaways for Public Health Researchers
For public health researchers and practitioners, the lessons from this paper are clear. First, when conducting or interpreting a meta-analysis, pay close attention to how the studies were selected and coded. A meta-analysis is only as good as the data it includes, so it’s important to ensure that the search was thorough and the coding was accurate.
Second, be cautious of meta-analyses that rely heavily on studies from top-tier journals or wealthy countries. While these studies are valuable, they may not reflect the full picture, especially in global health contexts where public health challenges can vary widely across regions.
Finally, consider partnering with a librarian or bibliometrician to help ensure that your search is as exhaustive as possible. These professionals have expertise in navigating the complex world of academic databases and can help you find studies that might otherwise be missed.
Join the Conversation
What do you think are the biggest challenges in conducting meta-analyses in public health? Have you encountered any of the issues mentioned in this blog in your own work? Share your thoughts in the comments below or join the conversation on social media using #MetaAnalysisBestPractices.
Empower Your Network – Subscribe and Share!
Unlock key insights with ‘This Week in Public Health.’ Subscribe for free and share to drive change as part of a dedicated community. If you liked this blog, please share it! Your referrals help This Week in Public Health reach new readers.
Leave a Reply