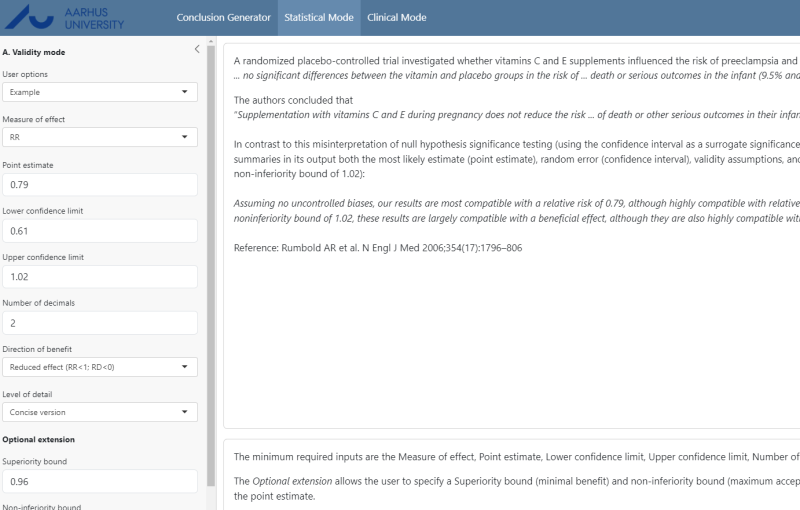
The Conclusion Generator: A Redundant Tool?
by Jon Scaccia August 12, 2024Look there was a time when I relied on online widgets instead of writing my own code. These tools were helpful, but there is something to much more fundamentally satisfying about getting deep into the data manipulation.
But not everyone is at that point in their journey. Enter the Conclusion Generator.
Why Should You Care?
Have you ever wondered if the conclusions drawn from scientific research truly reflect the data? Often, research findings are boiled down to whether they are “statistically significant” or not, but this can lead to misunderstandings.
For years, the scientific community has relied heavily on null hypothesis significance testing (NHST) to determine the validity of research findings. This method, while useful, has its flaws. One of the most significant issues is the misconception that if a result is not statistically significant (p ≥ 0.05), it means there is no difference between groups. Conversely, if a result is statistically significant (p < 0.05), it is often seen as inherently important and unbiased. This black-and-white thinking can be misleading and detrimental to scientific progress.
The Conclusion Generator aims to address these issues by focusing on the estimation and clinical relevance of research findings rather than simply on statistical significance.
Understanding the Conclusion Generator
The Conclusion Generator is a free online application designed to generate more nuanced conclusions for scientific papers. It uses the values and clinical interpretation of point estimates and confidence intervals to produce its outputs. The tool supports both relative and absolute measures of effect and offers two modes for interpretation:
- Statistical Mode: This mode provides a precise statistical interpretation of results. Users can specify superiority and noninferiority bounds, which help determine if a treatment is better than another or just not worse.
- Clinical Mode: This mode focuses on the clinical importance of the point estimate and confidence limits. Users define what they consider a clinically relevant effect, allowing the tool to generate conclusions that reflect real-world importance.
How It Works
The tool requires specific inputs from the user, including the point estimate, confidence limits, and the number of decimals for the output. Users also need to indicate the direction of a beneficial effect (e.g., whether a relative risk of less than 1 is beneficial). Based on these inputs, the Conclusion Generator formulates an interpretation that avoids the common pitfalls of NHST.
For example, consider a study testing a drug’s effectiveness in reducing infection risk. Suppose the relative risk (RR) is 0.50 with a 95% confidence interval of 0.10 to 2.58 and a p-value of 0.40. In that case, traditional NHST might conclude that the drug is ineffective because the result is not statistically significant. However, the Conclusion Generator might interpret this data differently, acknowledging the potential for substantial benefit or harm and the uncertainty involved.
Validating the Tool
The Conclusion Generator has been validated using both published and simulated data. In one case, it reinterpreted data from a study on vitamin C and E supplements during pregnancy. The original study concluded no significant differences between groups, but the Conclusion Generator highlighted that the data suggested a 21% reduction in the risk of serious outcomes – a potentially important finding that the NHST approach had downplayed.
Similarly, hypothetical scenarios from a randomized controlled trial of a drug to prevent wound infection were used to test the tool. The Conclusion Generator consistently provided interpretations that aligned with biostatistical and epidemiological recommendations, avoiding the binary trap of statistical significance.
Real-World Impact
So, why does this matter? Misinterpretations of research findings can lead to ineffective or even harmful public health policies and clinical practices. By providing a more accurate and clinically relevant interpretation of data, the Conclusion Generator can help ensure that decisions are based on a comprehensive understanding of the evidence.
This tool offers public health practitioners and researchers a way to enhance the accuracy and relevance of their work. By emphasizing estimation and clinical significance over NHST, the Conclusion Generator supports a more nuanced and informed approach to data interpretation.
Join the Conversation
What are your thoughts on the Conclusion Generator? Do you think this tool could change how research results are interpreted in your field? Share your experiences and impressions in the comments below or join the conversation on social media.
Editorial Review
I mean, couldn’t you just plug the same inputs into a large language model (LLM) and have it write the section for you? With the advancements in artificial intelligence, LLMs can indeed generate text based on given data inputs. However, not everyone is at the same point in their journey with these technologies. While LLMs are powerful tools, they require a certain level of familiarity and comfort with AI to be used effectively.
Moreover, LLMs might not always provide the specific clinical and statistical nuances needed for accurate scientific interpretation. This is where the Conclusion Generator have value. It is designed with a focused purpose: to mitigate common misinterpretations of research data by emphasizing estimation and clinical relevance. This tool provides a straightforward and user-friendly interface, making it accessible even to those who might not be comfortable using more complex AI tools.
So, while LLMs can be incredibly useful, tools like the Conclusion Generator offer a more specialized and tailored approach to interpreting research findings. It’s worth giving it a spin, so check it out here.
Be Part of the Change – Get Weekly Updates!
Stay informed and connected. Subscribe for free and share this blog to make a difference in public health with others.
Leave a Reply