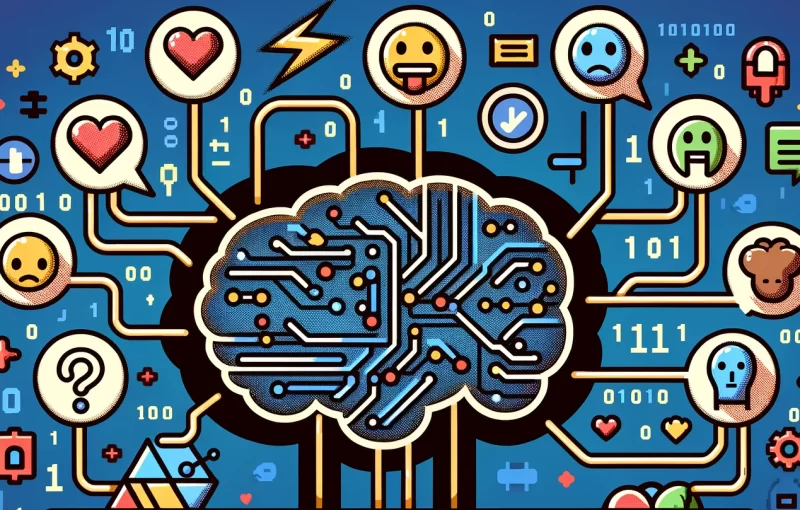
Explainable AI for Mental Health Detection: Illuminating the Path Forward
by Jon Scaccia June 3, 2024Artificial Intelligence (AI) is unlocking new frontiers in healthcare, particularly for mental health detection. As social media increasingly becomes a reflection of our inner worlds, researchers are harnessing its data to spot early signs of mental health conditions. This emerging field, known as Mental Illness Detection and Analysis on Social media (MIDAS), uses Natural Language Processing (NLP) and Machine Learning (ML) to parse through language patterns that may reveal early warning signs of disorders like anxiety, depression, or bipolar disorder.
While this technology promises groundbreaking benefits, it’s essential to make AI interpretable, particularly in sensitive domains like mental health. Research teams have been exploring how to balance the predictive power of deep learning models with the transparency that healthcare professionals require.
The Challenge of Interpretability in Mental Health AI
One recent research paper delves into this by systematically investigating explainable AI (XAI) methods to analyze mental disorders via language patterns in social media posts. They build BiLSTM (Bidirectional Long Short-Term Memory) models trained on hundreds of features like syntax, vocabulary, and emotions.
These interpretable features include:
- Syntactic Complexity: Sentence structures reveal linguistic diversity or rigidity.
- Lexical Sophistication: Sophisticated word choices can correlate with creativity, while simple words may reflect withdrawal.
- Readability & Cohesion: How easy is the text to understand, and how well do the ideas flow?
- Sentiment & Emotions: Detecting joy, sadness, fear, and other emotions that may align with various disorders.
The researchers then compared these models with a deep learning-based “black box” transformer to assess which model offers the best balance between accuracy and interpretability.
Multi-Task Learning and Interpretability Techniques
A significant challenge was making the models not only predict mental disorders with high accuracy but also explain why they made those predictions. To this end, the researchers employed two distinct explanation techniques:
- Local Interpretable Model-Agnostic Explanations (LIME): This method identifies specific features that most influenced the model’s decision.
- Attention Gradient (AGRAD): An attention-based method that highlights the words the model focused on during predictions.
Additionally, the researchers infused their AI with contextual information about emotions and personality traits to improve its interpretability and predictive power. They leveraged multi-task learning to combine these data streams, enriching their models to reveal deeper insights.
Key Findings and Insights
- Accuracy vs. Transparency: While the “black-box” transformer was more accurate in predicting mental disorders, the BiLSTM models were more transparent, offering crucial insights for practitioners to understand predictions.
- Emotion and Personality Data: By incorporating emotions and personality traits into the analysis, the models became more accurate and capable of explaining their predictions.
- Predictive Features: Stylistic, syntactic, and cohesion features were highly predictive for disorders like ADHD, anxiety, and depression.
The Road Ahead: Making AI Work for Mental Health
Explainable AI in mental health detection is not a mere technological feat but a critical ethical endeavor. Healthcare professionals need transparent models that align with their clinical expertise and patient communication. Moreover, patients must feel secure knowing why a model reaches its conclusions.
Discussion Questions (let us know in the comments!)
- How might explainable AI help destigmatize mental health issues through early detection and analysis?
- What ethical considerations should be addressed when leveraging social media data for mental health predictions?
Become a Health Innovator – Get Weekly Updates!
Join the forefront of public health innovation with “This Week in Public Health.” Each issue keeps you up-to-date with the latest in research, community health, and advocacy. This newsletter is more than just updates – it’s your tool for being an informed and active member of the health community. Subscribe for free and be part of a group dedicated to making a real difference in public health.
About the Author
Jon Scaccia, with a Ph.D. in clinical-community psychology and a research fellowship at the US Department of Health and Human Services with expertise in public health systems and quality programs. He specializes in implementing innovative, data-informed strategies to enhance community health and development. Jon helped develop the R=MC² readiness model, which aids organizations in effectively navigating change.
Leave a Reply