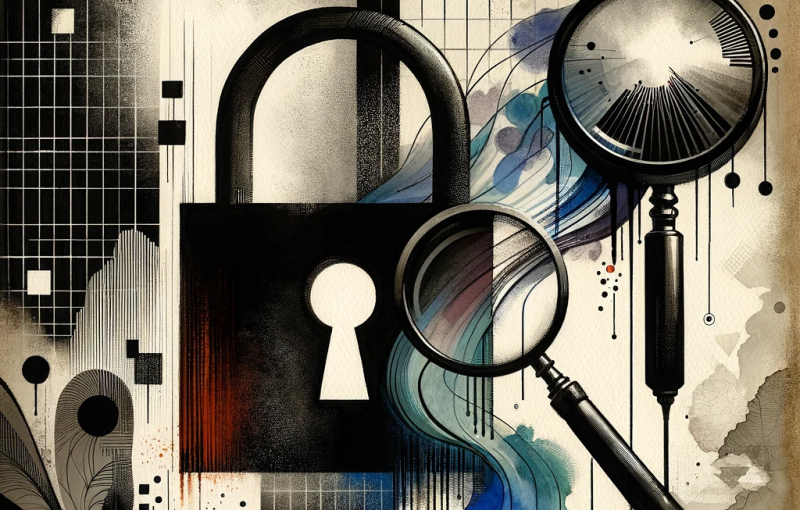
The Balance of Data Privacy and Utility in the 2020 US Census: A Closer Look at Disclosure Avoidance Techniques
by Jon Scaccia January 9, 2024The United States Census is a monumental task that has evolved since its inception, reflecting changes in society, technology, and governance needs. The article Confidentiality Protection in the 2020 US Census of Population and Housing delves into the complexities of protecting individual privacy while providing useful data from the 2020 US Census.
The Evolution of Data Privacy and the Adoption of Differential Privacy
In data privacy, especially in the decennial US Census, ensuring the confidentiality of respondents while providing useful data for countless applications has always been a paramount concern. Traditionally, the Census Bureau employed data swapping and suppression to protect individuals’ privacy. Data swapping involves exchanging certain attributes between respondents to reduce the risk of identification, while suppression involves not publishing certain details, especially in small population groups, to avoid revealing individual identities. These methods aimed to create a balance by making it difficult to attribute specific data to an individual without significantly compromising the data’s usefulness for analysis and decision-making.
However, as computational technology has advanced, so too have the methods that can be used to compromise data privacy. The rise of sophisticated data mining techniques and the availability of extensive external databases have made it easier to cross-reference and potentially re-identify individuals, even from anonymized datasets. This is particularly problematic for the Census, given its detailed breakdowns by geography and demographics. The increasing risk of identifying individuals from supposedly anonymous data has questioned the adequacy of traditional privacy protection methods.
In response to these challenges, the 2020 Census adopted a differential privacy framework, marking a significant shift in approach to confidentiality. Differential privacy is a mathematically rigorous method that provides a quantifiable measure of privacy. It works by adding controlled, random noise to the data to mask the contribution of individual respondents. The “noise” introduced is systematic and tuned to preserve the overall statistical characteristics of the data, such as means and variances, thereby maintaining its utility for public policy, research, and other applications.
Implications for Public Health Practitioners and Data Users
The shift to differential privacy in the 2020 Census marks a critical transition in how demographic data is handled, particularly impacting fields reliant on precise data, like public health. For practitioners in this field, understanding the mechanics and implications of noise addition under differential privacy is essential for effective planning, resource allocation, and policymaking.
Understanding Noise Addition in Differential Privacy:
At its core, differential privacy introduces calibrated “noise” to the data to mask individual contributions. This noise is not random but carefully calculated to maintain the overall structure and utility of the data. Here’s how it generally works at a macro level:
- Identifying Sensitive Data: Before adding noise, it’s crucial to identify which data is sensitive and at what granularity. In the case of the census, this might be individual responses or small group statistics that could reveal information about an individual.
- Choosing a Privacy Parameter (ε): Differential privacy is often characterized by a parameter epsilon (ε), which quantifies the privacy guarantee. A smaller ε provides stronger privacy but more noise, while a larger ε offers weaker privacy with less noise. Deciding on ε is a critical step as it defines the privacy-utility trade-off.
- Adding Noise Based on Data Sensitivity: Noise is then added to the data. The amount and type of noise depend on the data’s sensitivity and the chosen ε. For example, the Laplace or Gaussian mechanisms are common methods for noise addition, where the scale of the noise is proportional to the sensitivity of the function being computed (e.g., total population count) and inversely proportional to ε.
- Post-Processing for Consistency: After noise addition, the data might need adjustments to ensure consistency and usability, such as ensuring population counts don’t become negative or making sure the data aligns with known margins or totals.
What does this all mean?
- Planning and Resource Allocation: Public health initiatives often require data at a fine geographic level or for specific demographic groups to target interventions effectively. Differential privacy, while protecting individual data, might introduce uncertainty, particularly in sparse data areas or minority groups. This uncertainty can affect trend analysis, identification of health disparities, or resource allocation.
- Policy-Making and Public Trust: Policies based on demographic data must be robust and reliable. Introducing noise necessitates a more nuanced approach to interpreting data, understanding the possible range of error, and communicating these limitations in policy discussions. Maintaining public trust in the use of their data for decision-making is crucial, requiring transparency about the level of uncertainty in the data due to privacy protections.
- Adapting Analytical Techniques: Traditional data analysis techniques might not account for the noise introduced by differential privacy. Practitioners may need to adapt their methods, for instance, by using techniques robust to noise or by interpreting results with an understanding of the added uncertainty.
Balancing Data Utility and Privacy: A Key Challenge
The beauty of differential privacy lies in its flexibility and theoretical guarantees. It allows the Census Bureau to adjust the noise level based on the data’s sensitivity and the desired level of privacy protection. This means that for more sensitive or granular data, such as detailed geographic locations or smaller demographic groups, more noise can be added to ensure privacy. Conversely, less noise can be introduced for less sensitive data, preserving higher data utility. The end goal is a controlled trade-off between risk and utility, providing robust privacy protection while allowing for meaningful census data analysis.
Adopting differential privacy in the 2020 Census reflects a proactive approach to safeguarding privacy in an era of rapidly evolving data analysis capabilities. It’s a commitment to the responsible stewardship of personal data, ensuring that individuals’ privacy is protected under the highest standards of confidentiality. As we move forward, understanding and refining these techniques will be crucial in maintaining the trust and participation of the public, which is fundamental to the success of the Census and other data-informed initiatives.
While differential privacy offers a more robust protection mechanism, it does so by sacrificing some data granularity and accuracy. Understanding and navigating these trade-offs is crucial for data users, especially when employing the data for critical decisions in public health, policy making, and beyond.
Looking Ahead: Future of Data Privacy in Statistical Releases
As we look forward, the adoption of differential privacy in the 2020 Census sets a precedent for other statistical agencies and surveys. The ongoing challenge will be to refine these techniques to optimize the balance between privacy and data utility. Continuous dialogue between data users, statisticians, and privacy experts will be vital in shaping future approaches and ensuring that public trust in statistical data remains strong.
Conclusion: Engaging with the Full Article for a Deeper Dive
The article “Confidentiality Protection in the 2020 US Census of Population and Housing” is a rich resource for understanding the intricate balance of privacy and utility in large-scale data collection efforts. Readers are encouraged to engage with the original text to fully appreciate this topic’s complexities and nuances.
Further Reading and Exploration
To truly appreciate the advancements and challenges discussed in this blog, readers are encouraged to access the full article Confidentiality Protection in the 2020 US Census of Population and Housing.
Be the Health Change-Maker – Get Informed Weekly!
Step into the role of a public health change-maker with ‘This Week in Public Health.’ Each issue brings you closer to the heartbeat of community health, innovative research, and advocacy. It’s more than news; it’s a platform for transformation. Subscribe for free and join a community of informed individuals driving positive change in public health every week!
Leave a Reply