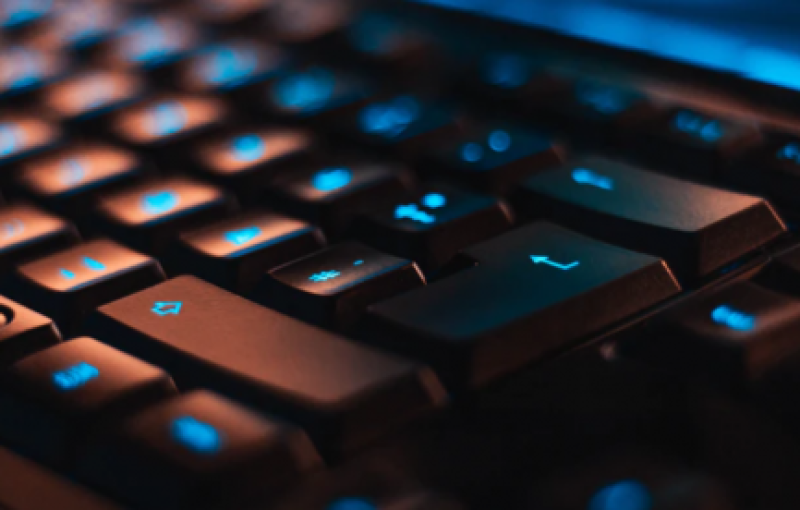
Reddit, Twitter, and more: August in Suicide Research
by Jon Scaccia August 27, 2021For August, we went a little deeper in the whole “discourse space” on suicide. In addition to the scan of the published academic literature and Twitter trends, we also pulled some data from Reddit. While the Reddit analysis is more of a work in progress, it shows some interesting insights into the types of things being shared online. Read on to see what trends are rising in prominence.
The academic literature
Like in previous posts, we pulled all articles from the last 31 days from Suicide, Suicide & Life-Threatening Behavior, Archives of Suicide Research, Suicidology Online, and Suicidologi. If you think we should be pulling from other journals, let us know in the comments. We then searched for any instance of “suicid*”. This yielded 362 articles across 210 journals, so pretty comparable to July’s article pull.
Words and Word Phrases
We first used the bag of words approach. This treats the words as meaningful in and of themselves, so a word that appears more frequently is likely to be more important (correcting for words like “and” & “the”). Click on the images below to see the word frequencies across abstracts in a few different formats. To editorialize, though, the network plot probably shows the most useful information.

Topic Clusters
If you haven’t read this column before, we use latent Dirichlet allocation to cluster the topics. This NLP method assumes that topics are composed of clusters of words, and then that articles are composed of clusters of topics. We can identify the clusters, then determine the words that make the cluster unique. Otherwise, we’d just be seeing the word “suicide” all over the place. Finally, we assign each article to its most likely cluster given its distribution of words.
The two figures show distributions of these articles. The figure at the right shows how articles are distributed in two-dimensional space. It’s pretty but doesn’t provide a lot of intuitive understanding of the research.
Better is the figure below. This shows the topic phrases that distinguish the clusters and the number of articles that talk about that topic. So, the #1 topic deals with mental health characteristics. Another topic deals with firearm mortality. And there were a few articles in Polish!

Because we like to look at the relationships between topics, we computed the correlations between them. Despite the visual differences, these correlations are all around 0.2, which is generally what we find when we compute these. And that makes sense! If the correlations were really strong, then the topic clusters wouldn’t have emerged as independent of one another.

Key Articles
A buddy let me know that he found excel files better than .pdfs because he could add them into his ongoing reading list. So, here’s an excel download of the review articles over the past month.
And, here is a list of the articles that were most representative of each topic. This also includes topic summaries, which we derive by finding the sentences that are most representative of each topic.
Let’s take another short dive into Twitter to see what people are saying in tweets about #suicide. Twitter’s API has a rate cap that limits the number of tweets you can pull (18000) and the length of time you can pull them from (one week).
Using a similar method to above, we clustered the tweets into topics. We can see that in addition to the mental health topics, we’re picking up tweets from the August 26th bombing of the Kabul Airport.

We also did a quick sentiment analysis of the tweet text. Words don’t just communicate ideas. They can express emotion. And, emotion is a critical aspect of how people relate to one another. Sentiment Analysis is an NLP technique that looks at the emotional content behind the words.
Sentiment analysis is well-established in customer service. It can be an essential technique to learn how customers perceive the value of a product. Many research studies have explored the potential of these methods in behavioral health. We used the NRC lexicon to identify specific emotions communicated in these tweets. We removed the word “suicide” from this analysis.

Reddit: A work in progress
We also tried to pull from the subreddit r/SuicideWatch to examine the chatter going on there. This mission of this subreddit is, “Peer support for anyone struggling with suicidal thoughts.”
We pulled the titles of 992 threads and then did a similar clustering. Instead, we show the words most predictive of each topic, instead of the words that distinguish each topic.

We ran up against a much tighter rate limit with Reddit’s API. Because of that, getting the comments under each thread ran us up against a cap. While we ended up pulling about 10 threads with comments, the text analysis we did wasn’t really that informative, so it’s a little bit of back to the drawing board.
What’s Next
Think we should add another data source to the mix? Let us know in the comments and we’ll try to make it happen.
Don’t forget to check out our totally free open search to get the latest trends across the biomedical and social sciences. Did we say it’s free? And follow us on Twitter and all that other jazz.

Leave a Reply