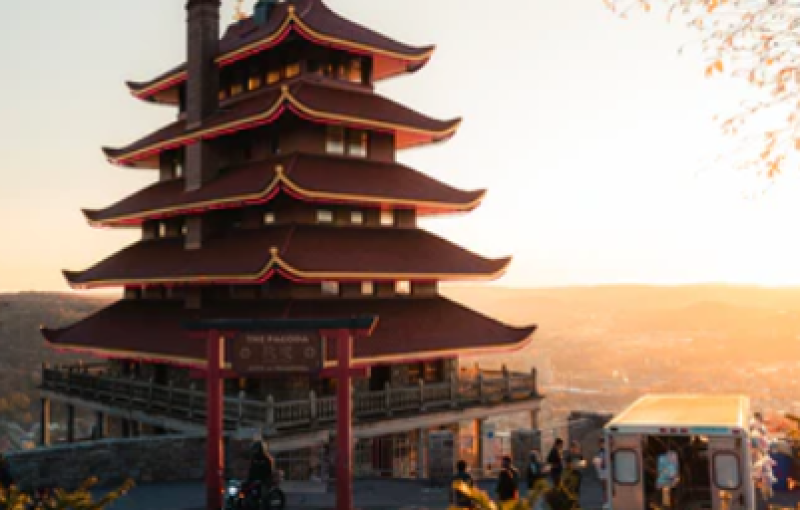
Income Inequality and Educational COVID Response
by Jon Scaccia June 23, 2021Over a year out, how did schools do in responding to COVID? The truth is we don’t quite know yet; maybe it was good, maybe it was terrible? I based a large part of my failed candidacy for my local school board on the premise that we needed to find out the impact so we can adjust accordingly. Evaluation of academic and SEL outcomes is going to take years. In the meantime, my colleague Allison Herb and I were interested in seeing whether there were any proxy measures of how school districts, specifically school boards, responded to the crisis.
For the SCRA biennial conference, we took a look at the public record of school policy decision making, the minutes. We pulled all the school board minutes for all the districts in our county (Berks, PA) going back to roughly the beginning of the pandemic (February 2020). We then pulled the Gini Coefficient for each school district.
The Gini Coefficient is a measure of income inequality, meaning that wealth is unevenly distributed. We pulled this data from Policy Map. We could have used other measures like poverty rate or proportion of students on free lunch, but given the exploratory nature of our study, we decided to start here.
There’s a lot of variability in how thoroughly different districts documented their board minutes. Some had regular meetings, others not as much. We pulled every mention of COVID, Coronavirus, and Pandemic, and then computed what percent of the discussion focused on these. As seen below, most of the districts averaged around 1%, with two going over 4%.

We then ran an ANOVA seeing how the proportion of discussion related to different levels, as defined as policy map. The result was insignificant, but not widely so; F(4,12), 2.18, p = 0.13. We plotted out the specific results below by community.

What’s next?
This was just an exploratory study, but there’s a lot more we can do to make this a stronger analysis, including more robust data extraction from the minutes and adding a few better SDOH measures.
Download our poster below!
Leave a Reply