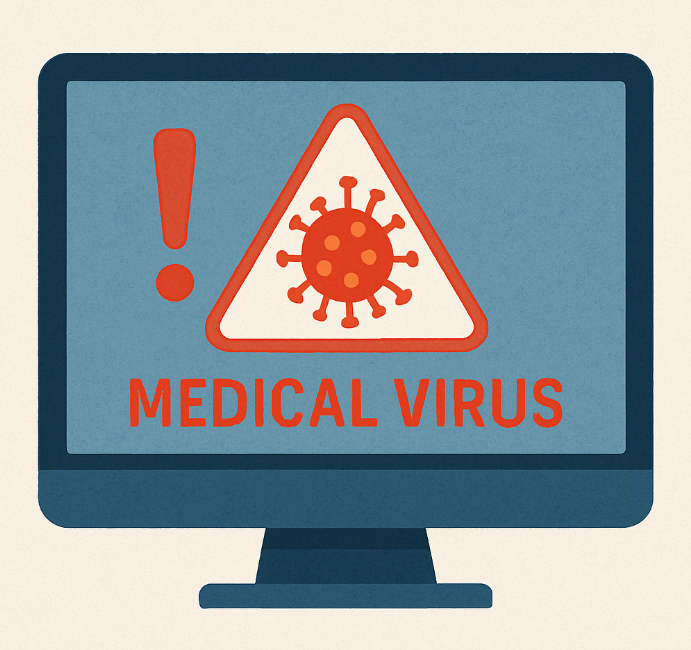
Why 89% of Pandemic Search Data Predicts Outbreak Trends
You’re at home. You hear someone at work tested positive for COVID-19. Without even thinking, your fingers tap out “COVID symptoms” into the search bar. You’re not alone—millions of others are doing the same. But what if those frantic searches could do more than just offer answers? What if they could help predict the next outbreak?
A recent study from China suggests exactly that. By using a mathematical model built on three years of COVID-era search and case data, researchers found a dynamic, feedback loop between what people search for and how epidemics unfold. In other words: the more people search, the more cases might be detected—and the more cases there are, the more people search.
It’s not just correlation. It’s causation in motion.
From Curiosity to Contagion: Why This Study Matters
Traditional epidemic surveillance has a serious lag problem. Reporting delays, undercounting, and bureaucratic bottlenecks often mean that by the time health authorities know something’s wrong, the situation is already out of hand.
But search engines? They offer something public health desperately needs: speed.
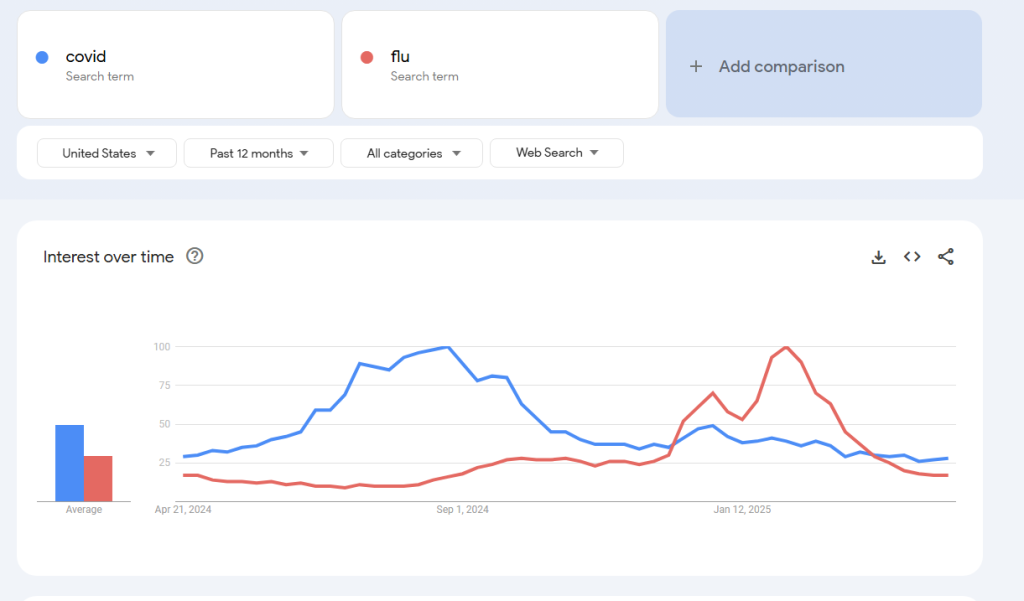
Using Baidu’s massive search engine data (think “Google of China”) and official COVID-19 case reports, the study built a non-linear differential equation model. What’s that in plain language? A math formula that doesn’t just draw a straight line between cases and clicks. Instead, it accounts for how people react over time—and how that reaction loops back into real-world outcomes.
The result? A model with a correlation coefficient of 0.89 between cumulative COVID-19 cases and search behavior—a near-perfect alignment. When the researchers segmented the data by year and removed noise (like the Omicron spike in 2022), the model’s predictive power soared to 0.98.
That’s not just impressive. That’s a wake-up call for public health systems still relying on slow-moving traditional data.
How It Works: A Feedback Loop Between Search and Spread
Here’s the fascinating part: The researchers didn’t just show that search behavior reflects what’s happening—they showed it might drive what happens next.
The model reveals that as confirmed COVID-19 cases rise, search behavior spikes. That’s expected. But in turn, this wave of searching also prompts people to seek testing, report symptoms, and take action—leading to more confirmed cases. It’s a self-reinforcing cycle.
Think of it like this: search engines are both the mirror and the magnifying glass. They reflect rising anxiety—and can amplify public response.
The researchers even mapped this effect over three pandemic phases:
- Latent phase: Low case counts, but rising searches—early warning signs.
- Outbreak phase: Search behavior explodes, tightly linked to a surge in confirmed cases.
- Decline phase: Searches drop off as the pandemic recedes.
This temporal sensitivity is crucial. It means search data could help public health authorities move from reactive to proactive strategies.
From Clicks to Crisis Management: Real-World Impact
Let’s say you’re a health department analyst. One morning, you notice a spike in searches for “loss of smell” and “COVID testing near me” in a specific zip code. The new model suggests this isn’t just noise. It could mean that in a few weeks, that area might see a surge in cases.
That gives you time—time to target testing, scale up communication, and reinforce local mitigation measures before hospitalizations spike.
It’s like weather forecasting, but for viruses.
This approach also helps reduce blind spots. People may search before they feel comfortable seeing a doctor. They may search out of fear, curiosity, or when they first notice symptoms. That search activity becomes a digital smoke signal—one that the model can now quantify and use.
The Downside: Search Engines Are Biased, Too
Of course, search data isn’t perfect. Search engines use personalized algorithms. What you see isn’t always what your neighbor sees. This can lead to filter bubbles, misinformation, and uneven data quality.
The study acknowledges this. Future models may need to weight search terms differently, account for media coverage surges, or distinguish between panic-induced spikes and genuinely informative trends.
Still, as the study shows, these signals—even with noise—can be sharpened into powerful early warning tools.
What’s Next: Beyond COVID-19
The biggest value of this research isn’t just for COVID-19. It’s that the same modeling approach could apply to other infectious diseases—flu, RSV, or even monkeypox. It gives us a flexible framework that can adapt across outbreaks, cultures, and technologies.
Imagine integrating this model with Google Trends, Twitter data, or even symptom-tracking apps. You’d have a real-time dashboard of public concern—filtered through mathematical precision.
Let’s Talk: What Could This Look Like in Your Community?
This research changes the way we think about outbreak surveillance. But what about your own neighborhood?
- Would real-time search data help your health department stay ahead?
- How could communities use this information to target outreach more effectively?
- What ethical guardrails should we build to prevent misuse of search-based surveillance?
Don’t Let Science Pass You By
⚠️ Public health breakthroughs and threats are happening fast.
Missing one update could mean missing the chance to act, advocate, or lead. Stay sharp, stay informed—with our weekly science brief, trusted by changemakers.✅ Subscribe now—it’s free and essential.
📣 Share this blog to grow the circle of informed action.