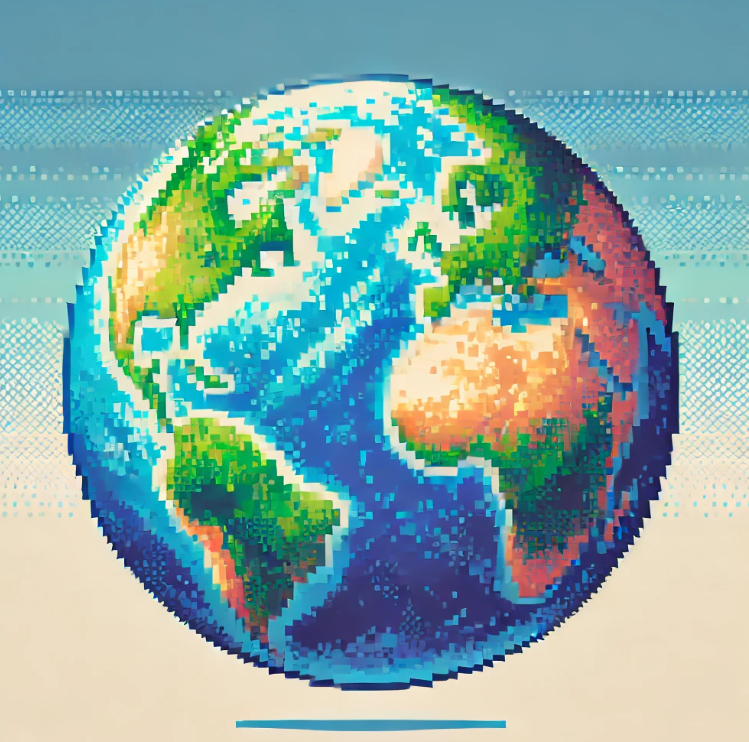
Are Clinical Trial Results Actually Useful for You?
A breakthrough cancer drug shows promising results in a clinical trial. Doctors and policymakers are eager to adopt it.
But there’s a catch—the study was conducted on a group of patients who are younger, healthier, and more affluent than the population it’s meant to serve. Will the treatment work just as well for older adults or individuals with pre-existing conditions? Historically, answering this question has been tricky. But new statistical methods—transportability and generalizability analyses—are reshaping how we apply medical research beyond the controlled world of clinical trials.
The Problem: Research That Stays in a Bubble
Clinical trials are the gold standard for testing new treatments, but they often suffer from low external validity—meaning their findings may not hold true for the broader population. This is because trials recruit specific participants who meet strict eligibility criteria, often excluding people with multiple health conditions, those from diverse racial or socioeconomic backgrounds, or older adults.
For years, the focus of research has been on making sure studies are internally valid—that is, ensuring the results are accurate within the trial itself. But what happens when a treatment moves from the lab to the real world? If the trial population doesn’t match the people who will actually receive the treatment, medical decisions based on the trial may be flawed.
The Solution: Transportability and Generalizability Analyses
To bridge this gap, researchers are turning to causal inference methods that assess how well study findings apply to different populations.
- Generalizability analysis extends results from a study sample to a larger target population. This is useful when a clinical trial is conducted on a small, selective group but needs to be applied to a broader demographic.
- Transportability analysis goes one step further—it applies findings from one study to an entirely different setting. For instance, a drug trial conducted in the U.S. might need to be adapted for patients in sub-Saharan Africa, where health conditions and risk factors may differ.
These methods adjust for effect modifiers—factors like age, gender, or disease severity that could alter how a treatment works in different populations. By reweighting or recalibrating the data, transportability and generalizability analyses provide more relevant, actionable insights.
Real-World Applications: How These Methods Are Changing Public Health
A recent systematic review examined 68 studies that applied these methods in real-world datasets. The findings were promising:
- Better Decision-Making: Transportability analysis helped policymakers adapt trial results for underrepresented groups, improving equity in medical recommendations.
- Improved Trial Design: Researchers used these methods to identify which patient characteristics influenced treatment outcomes, helping refine eligibility criteria for future studies.
- Cost Savings: By making existing trial data more applicable, these methods reduced the need for expensive, redundant studies in new populations.
One notable example was a study that applied transportability analysis to understand whether a hypertension treatment tested in a wealthy, urban population would be just as effective in rural communities with different dietary and lifestyle factors. The results suggested modifications were needed—without transportability analysis, doctors might have recommended an ineffective treatment.
What’s Next? Overcoming Challenges
Despite their potential, transportability and generalizability analyses aren’t yet widely adopted. Several challenges remain:
- Lack of Standardization: Reporting quality varies, making it difficult to compare studies.
- Data Access Issues: These methods require detailed patient data, which is often restricted due to privacy concerns.
- Limited Software Options: Few user-friendly tools exist for researchers who lack advanced statistical training.
Fortunately, momentum is building. Organizations like the National Institute for Health and Care Excellence (NICE) are incorporating these analyses into their real-world evidence frameworks, and new statistical software is being developed to make these techniques more accessible.
Join the Conversation
As clinical research evolves, so must our ability to interpret and apply its findings. How do you think transportability and generalizability analyses could impact medical decision-making in your field? Have you encountered situations where clinical trial findings didn’t align with real-world patient outcomes? Let’s discuss—share your thoughts in the comments or on social media!
Be a Changemaker – Join Us Today!
Stay ahead of the latest public health developments. Subscribe for free now to receive weekly updates that empower you to lead and create impact.
💡 Every moment counts! Share this blog and invite others to take action for a healthier tomorrow.