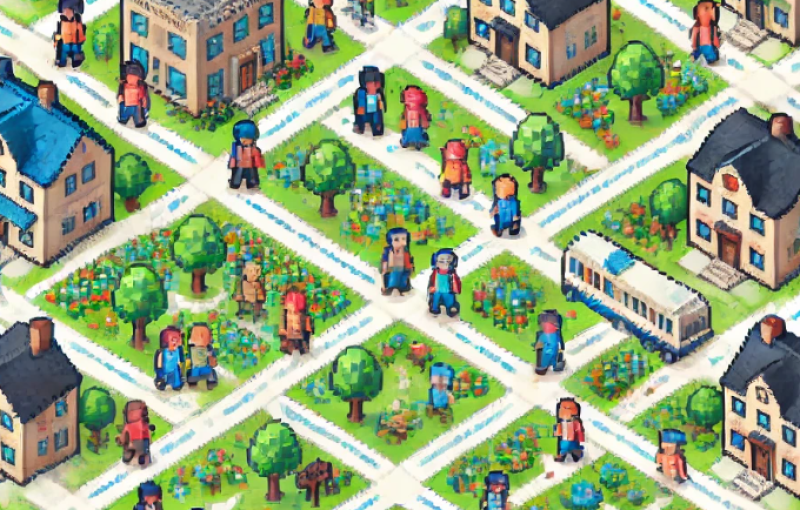
Agent-Based Modeling for Public Health Initiatives
by Jon Scaccia December 10, 2024Agent-based modeling (ABM) has emerged as a transformative tool for understanding complex systems in public health. Imagine a virtual world where individual “agents” (such as people, households, or organizations) interact based on set rules. These interactions simulate real-life dynamics, shedding light on patterns that drive health outcomes. But ABM isn’t just for researchers; it has real-world applications for professionals administering public health and social services. By using ABMs, we can explore how different interventions might play out across communities, identify the most effective strategies, and even spot unintended consequences before they happen.
What is Agent-Based Modeling, and Why Does It Matter?
ABM allows public health professionals to “test-drive” interventions in a controlled, virtual environment. Each agent in an ABM simulation acts independently, with its behaviors influenced by personal and environmental factors. Through this setup, ABMs reveal how seemingly minor individual actions can produce larger community-level trends. This “bottom-up” approach contrasts with traditional models that often oversimplify interactions within a population.
Consider an ABM that simulates smoking behaviors in a community. Agents might vary in age, income, and social habits, all factors that affect smoking. By programming agents with these characteristics, the ABM can help public health professionals see how social influences, policies, and even community resources might change smoking rates over time.
This insight is invaluable, especially for professionals designing social services, as it allows them to tailor interventions to specific community needs, monitor ripple effects across populations, and ultimately maximize positive outcomes.
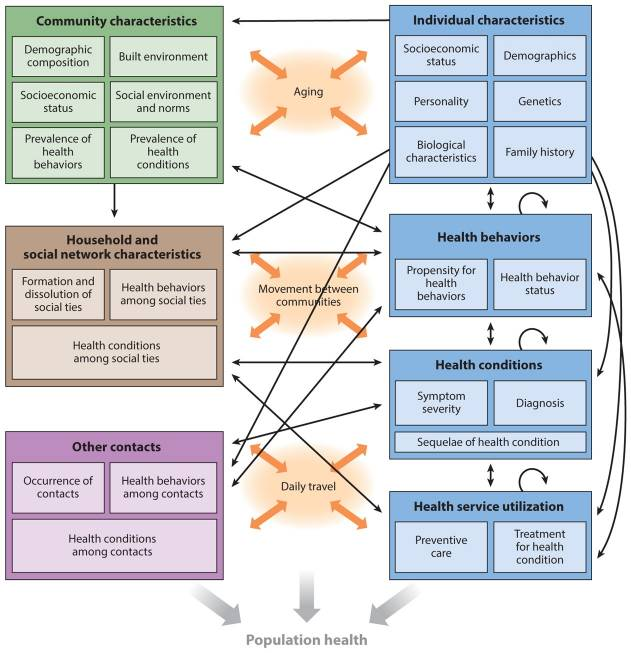
Applications of ABM in Public Health: From Disease Control to Health Behaviors
ABMs have proven effective across several public health areas, from infectious disease management to tackling complex social health issues.
1. Infectious Disease Control
ABMs gained prominence in infectious disease modeling. During pandemics like COVID-19, ABMs helped public health officials understand the spread of disease and the impact of measures like social distancing and vaccination. By examining different “what-if” scenarios, such as increasing vaccination rates or enforcing mask mandates, ABMs can guide response strategies for future outbreaks. These insights help public health teams allocate resources, plan containment strategies, and predict how policies might impact various population segments.
2. Managing Noncommunicable Diseases
While infectious disease models often receive the spotlight, ABMs are equally powerful for chronic health challenges. Take obesity, for example researchers can simulate how community-based exercise programs or improved access to healthy food might affect obesity rates. This allows policymakers to visualize long-term outcomes and better allocate resources for prevention. Additionally, it underscores the importance of targeting social influences on health, showing how peer behaviors can affect an individual’s habits.
3. Influencing Health Behaviors
Public health campaigns on smoking, alcohol use, and physical activity benefit immensely from ABMs. In smoking prevention, for instance, ABMs allow researchers to see how individuals react to policy changes, like increased taxes on tobacco products. These models can also simulate peer influence and the impact of different socioeconomic backgrounds, highlighting the need for targeted interventions to reduce smoking rates in specific communities. By examining how these factors intersect, ABMs offer a nuanced view that enhances policy effectiveness.
4. Social Epidemiology and Health Equity
ABMs can also tackle issues in social epidemiology, including violence prevention and incarceration. For instance, a model might simulate how neighborhood characteristics like crime rates and access to mental health services affect violence. The model could explore the potential impact of introducing community programs to strengthen neighborhood cohesion and reduce violence. This application is critical for professionals looking to address health disparities and improve social determinants of health, helping to design interventions that work in complex, real-world environments.
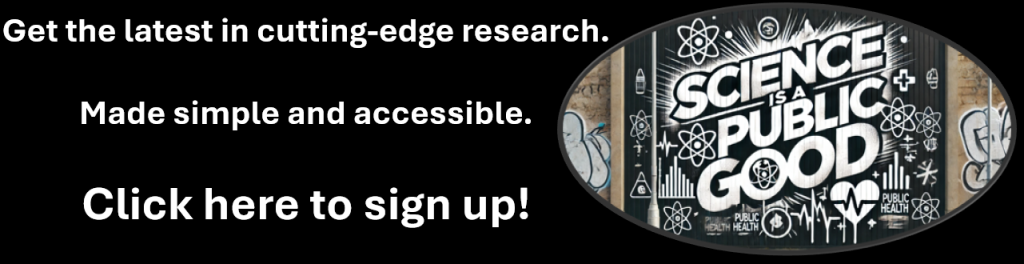
Strengths of ABM: Making Smarter Public Health Decisions
One of ABM’s biggest strengths is its flexibility. Public health professionals can customize ABMs to reflect local community characteristics, capturing diverse needs and preferences. Unlike one-size-fits-all approaches, ABMs empower professionals to see how different scenarios play out in their communities before launching a program.
Another advantage is ABM’s ability to highlight causal mechanisms, showing how individual choices ripple out to affect broader outcomes. This ability to “zoom in” and “zoom out” on health behaviors allows public health teams to target root causes of health issues more precisely.
ABMs also allow for virtual testing of interventions, making it possible to “replay” scenarios and refine strategies. This virtual testing is invaluable when budgets are tight, as it allows professionals to prioritize initiatives that yield the best results.
Limitations and Challenges in ABM for Public Health
While ABMs offer exciting opportunities, they’re not without challenges. These models require accurate data to ensure reliable results, and data gaps can limit the model’s accuracy. Another challenge is simplifying complex systems without sacrificing realism; if a model is too simple, it may miss key factors, while overly complex models may be hard to interpret.
Despite these hurdles, the future of ABM in public health is promising. As more data becomes available, ABMs will likely grow more accurate and easier to apply to real-world scenarios.
Actionable Insights: Using ABM to Improve Social Services and Public Health Programs
- Tailor Interventions to Community Needs
ABMs help identify which interventions are most effective in specific communities. Before launching a new program, consider using ABMs to assess how they might interact with existing community factors, such as social norms or economic conditions. - Test Multiple Scenarios for Optimal Impact
With ABMs, public health professionals can simulate various intervention scenarios. For instance, if your goal is to reduce obesity, test combinations of policies, like improving walkability and increasing access to healthy foods, to determine which works best together. - Evaluate Unintended Consequences
Every intervention has the potential for side effects. ABMs allow professionals to anticipate unintended consequences—like shifting problems to other areas—enabling adjustments before implementation. - Involve Stakeholders in Model Design
Engage local stakeholders in the development of ABMs. Their insights ensure that the model reflects community realities, and they can offer feedback on potential interventions. This collaborative approach builds trust and improves the model’s relevance.
Join the Conversation
How do you think ABM could benefit your community’s public health challenges? Are there programs in your area that could be more effective with ABM insights? Share your thoughts in the comments!
Bonus!
Here’s a git repo for an R package to implement ABM for epidemics.
Empower Your Network – Subscribe and Share!
Unlock key insights with ‘This Week in Public Health.’ Subscribe for free and share to drive change as part of a dedicated community. If you liked this blog, please share it! Your referrals help This Week in Public Health reach new readers.
Leave a Reply